“AI Is Getting Better at Mind-Reading” is how The New York Occasions places it.
The precise research, “Semantic reconstruction of continuous language from non-invasive brain recordings” (by Jerry Tang, Amanda LeBel, Shailee Jain & Alexander G. Huth of the College of Texas at Austin), printed in Nature Neuroscience, places it this fashion:
We introduce a decoder that takes non-invasive mind recordings made utilizing useful magnetic resonance imaging (fMRI) and reconstructs perceived or imagined stimuli utilizing steady pure language.
In the end, they developed a means to determine what an individual is considering—or not less than “the gist” of what they’re considering—even when they’re not saying something out loud, by taking a look at fMRI information.
Evidently such findings might need relevance to philosophers working throughout a spread of areas.
The scientists used fMRIs to report blood-oxygen-level-dependent (BOLD) alerts of their topics’ brains as they listened to hours of podcasts (like The Moth Radio Hour and Modern Love) and watched animated Pixar shorts. They then needed to “translate” the BOLD alerts into pure language. One factor that made this difficult is that ideas are quicker than blood:
Though fMRI has wonderful spatial specificity, the blood-oxygen-level-dependent (BOLD) sign that it measures is notoriously gradual—an impulse of neural exercise causes BOLD to rise and fall over roughly 10 s. For naturally spoken English (over two phrases per second), which means every mind picture might be affected by over 20 phrases. Decoding steady language thus requires fixing an ill-posed inverse drawback, as there are lots of extra phrases to decode than mind photos. Our decoder accomplishes this by producing candidate phrase sequences, scoring the chance that every candidate evoked the recorded mind responses after which selecting the right candidate. [references removed]
They then skilled an encoding mannequin to match phrase sequences to topics’ mind responses. Utilizing the information from their recordings of the themes whereas they listened to the podcasts,
We skilled the encoding mannequin on this dataset by extracting semantic options that seize the which means of stimulus phrases and utilizing linear regression to mannequin how the semantic options affect mind responses. Given any phrase sequence, the encoding mannequin predicts how the topic’s mind would reply when listening to the sequence with appreciable accuracy. The encoding mannequin can then rating the chance that the phrase sequence evoked the recorded mind responses by measuring how effectively the recorded mind responses match the expected mind responses.
There was nonetheless the issue of too many phrase prospects to feasibly work with, so they’d to determine a approach to slim down the interpretation choices. To do this, they used a “generative neural community language mannequin that was skilled on a big dataset of pure English phrase sequences” to “limit candidate sequences to well-formed English,” and a “beam search algorithm” to “effectively seek for the almost certainly phrase
sequences.”
When new phrases are detected based mostly on mind exercise in auditory and speech areas, the language mannequin generates continuations for every sequence within the beam utilizing the beforehand decoded phrases as context. The encoding mannequin then scores the chance that every continuation evoked the recorded mind responses, and the… almost certainly continuations are retained within the beam for the subsequent timestep.
They then had the themes hear, whereas present process fMRI, to podcasts that the system was not skilled on, to see whether or not it might decode the mind photos into pure language that described what the themes had been considering. The outcomes:
The decoded phrase sequences captured not solely the which means of the stimuli however usually even actual phrases and phrases, demonstrating that fine-grained semantic data might be recovered from the BOLD sign.
Listed here are some examples:
Element of Determine 1 from Tang et al, “Semantic reconstruction of steady language from non-invasive mind recordings”
They then had the themes bear fMRI whereas merely imagining listening to one of many tales they’d heard (once more, not a narrative the system was skilled on):
A key job for mind–laptop interfaces is decoding covert imagined speech within the absence of exterior stimuli. To check whether or not our language decoder can be utilized to decode imagined speech, topics imagined telling 5 1-min tales whereas being recorded with fMRI and individually informed the identical tales exterior of the scanner to supply reference transcripts. For every 1-min scan, we accurately recognized the story that the topic was imagining by decoding the scan, normalizing the similarity scores between the decoder prediction and the reference transcripts into chances and selecting the almost certainly transcript (100% identification accuracy)… Throughout tales, decoder predictions had been considerably extra much like the corresponding transcripts than anticipated by probability (P < 0.05, one-sided non-parametric check). Qualitative evaluation reveals that the decoder can recuperate the which means of imagined stimuli.
Some examples:
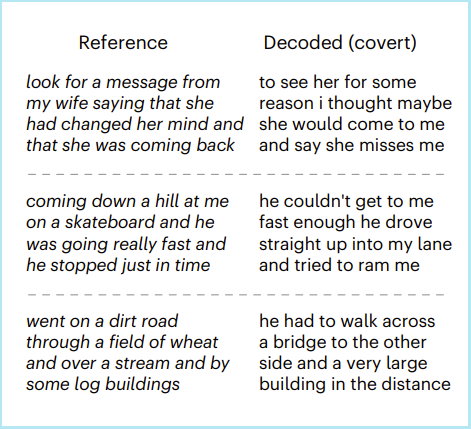
Element of Determine 3 from Tang et al, “Semantic reconstruction of steady language from non-invasive mind recordings”
The authors word that “topic cooperation is at the moment required each to coach and to use the decoder. Nevertheless, future developments may allow decoders to bypass these necessities,” and name for warning:
even when decoder predictions are inaccurate with out topic cooperation, they may very well be deliberately misinterpreted for malicious functions. For these and different unexpected causes, it’s essential to lift consciousness of the dangers of mind decoding know-how and enact insurance policies that defend every individual’s psychological privateness.
Observe that this research describes analysis carried out over a yr in the past (the paper was simply printed, however it was submitted in April of 2022). Given the obvious tempo of technological developments we’ve seen just lately, has a yr in the past ever appeared to this point prior to now?
Dialogue welcome.
Associated: Multimodal LLMs Are Here, GPT-4 and the Question of Intelligence, Philosophical Implications of New Thought-Imaging Technology.